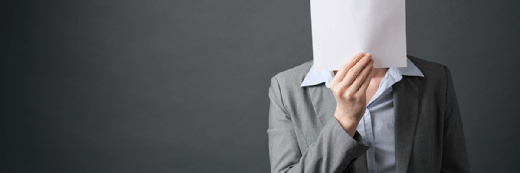
Data anonymization best practices protect sensitive data
See how data anonymization best practices can help your organization protect sensitive data and those who could be at risk if that data identified them.
Securing personally identifiable information is increasingly challenging for many organizations. As the amount of worldwide data increases, so does the number of rules, laws and policies to govern and protect it. This ever-growing volume of regulatory policies can bewilder even the savviest data privacy and legal professionals.
One of the top ways organizations can ensure security and privacy for personally identifiable information (PII) is following data anonymization best practices.
What is PII?
At a high level, PII is data that can be used on its own or combined with other information to uniquely identify a person. The goal of governmental and industry PII regulations is to define and enforce policies to keep that information private.
Two examples of well-publicized governmental regulatory frameworks that have defined a set of privacy policies for PII are the European Commission's GDPR framework and the California Consumer Privacy Act (CCPA). Infringement fines from both governmental agencies can be extensive. The GDPR levied over $126 million in financial penalties from its inception to January 2020, and CCPA fines range from $2,500 to $7,500 per record.
The challenge with identifying PII is that dozens of data elements are potential personal identifiers. In addition, government websites like the GDPR's information portal that provide PII data descriptions and examples use language that is intentionally vague as to not limit data elements to a predefined set. Because each of the regulatory frameworks may provide different interpretations of PII, it is best to consult an expert in that specific set of privacy controls.
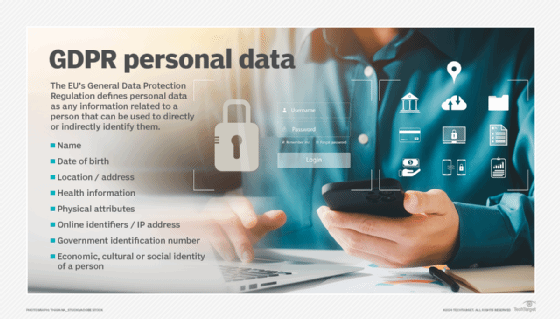
Data anonymization
From a PII perspective, Data anonymization is the process of obscuring information in a manner that prevents it from uniquely identifying an individual. Anonymization reduces the risk of accidental disclosure of PII data, and if a data breach does occur, the stolen information will be of no use to attackers.
As far as meeting regulations, anonymized data is considered non-personal data as individuals cannot be identified by it.
The ultimate goal of anonymization is to remove the chances of PII exposing an individual while retaining the value of the information to the organization. There is a fine line between anonymizing data and destroying its ability to provide meaningful business insights.
Data anonymization key to PII privacy
To properly protect PII data, businesses must develop a strategic program that defines the roles, rules, processes and best practices the organization will follow to ensure the safety, quality and proper use of its PII data. The program's goal is to provide a blueprint of controls that ensures the effective management of PII data at the enterprise level.
The inputs are standard IT community data anonymization best practices as well as corporate, industry-specific and governmental regulatory framework specifications and controls. The program should be a joint business and IT initiative with both departments playing equally important roles.
One of the outputs should be a documented a set of technology-agnostic controls that have universal application across the organization. Those controls must include a set of robust data anonymization policies and procedures.
A crucial component of protecting any sensitive information is to develop an enterprise-wide awareness program. IT departments can't protect PII on their own. All business and operational groups that interact with PII must become actively involved, and management buy-in is essential.
You can't anonymize data you don't know about
Before developing policies and procedures, buying products or implementing manual data anonymization processes, identify all potential PII data elements in your organization. The larger your environment, the more susceptible your organization will be to storing unidentified PII data.
This isn't an easy task. Most data including PII doesn't sit idle. Once a data element is created, it quickly spreads to reports, dashboards and other data stores across an organization.
Ensuring a person's continuous anonymity throughout an enterprise is inherently fluid by its nature. In other words: things change. Data audits and continuous feedback from IT and business personnel that interact with PII will help to identify potential issues.
Data anonymization products
From products that specifically focus on data anonymization best practices to enterprise-wide offerings that provide a wide range of data security features, there is wealth of software solutions available. The larger an organization is, the more important these tools become. Based on the amount of data your organization stores, you may need to purchase a product or two to properly identify and safeguard sensitive PII data assets.
There is a broad spectrum of data anonymization products that are available. In addition, some existing data storage platforms inherently provide anonymization features. As an example, many leading databases provide anonymization components such as encryption as part of their base software stack. There are also products that specialize in anonymizing data residing in a wide range of data stores that include cloud and on-premise databases and flat files.
To correctly select and implement the most appropriate PII identification and data anonymization software for an organizations, technology pros must create and execute a well-thought-out, detailed analysis of competing offerings.
Here is a quick list of general recommendations to help you jumpstart your analysis:
- Shops evaluating PII data identification and anonymization products should follow a standardized product evaluation methodology to facilitate the selection process. Evaluation best practices include selecting the appropriate evaluation team, performing a thorough needs analysis and creating a robust set of weighted evaluation metrics. Use the evaluation metrics to create a vendor shortlist and execute a deep-dive comparison of the remaining vendors.
- Understand your business needs. What are you trying to accomplish? Are you looking for an offering that focuses specifically on data anonymization, PII data identification or a platform that provides a much wider scope of features and functionality?
- Use peer review websites, such as Gartner Peer Insights, for community review of different offerings.
- Visit vendor websites and IT community discussion forums. Vendors often purchase Gartner's Vendor Magic Quadrants and make them available to the public, which may help narrow down your vendor shortlist.
- Identify if the vendor supports cloud, on premises or both environments, depending on your current and expected future needs.